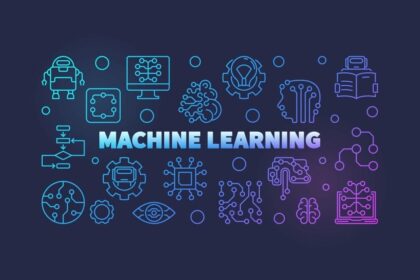
As I start writing this piece I get prompted if I have enabled the application on suggestions to help me with the same or on ways to complete a particular sentence or in ways to redefine my thought in a better way. In the morning, I was sending a mail for my tax filing I was getting prompted on how to complete my mail. I was searching for a car cover in the morning on one of the ecommerce apps and I was filled with suggestions even after I selected a product. The same happens when I order regular groceries on a different app which reminds me before I wind up if I have missed any of my usual purchase items and so on. These are all some of the few examples that we notice where ML/AI has already become part of our lives. There are hundreds of places where such situations are already happening which aren’t visible to us.
If we look at us humans, we learn things through neural networks which are formed in our brain. Once humans figured out how our neural networks worked, we tried to create similar ones on machines so that they could mimic learning in ways similar to the way that we do. Machine learning was born out of this endeavor. So basically, ML is an application of AI (Artificial Intelligence) in which the system automatically improves with experience like what humans do without the need to be specifically programmed to do these improvements. So, a well written ML algorithm will access data, analyze it and use it to improve its own performance. Artificial neural networks are usually trained by what is often called as a epoch. Epoch is the system very similar to human learning in which each data point is presented only once to the system and after this the system performs the task.
ML usually follows three different types of learning:
1. Supervised learning
This works by comparing the real time output with the desired output which has been provided and creates an error margin as feedback to improve the performance. This type of learning is usually used for tasks like classifications, approximations, identifications, optimizations and so on. This learning is also called as closed loop feedback system where the error measure guides the learning process.
2.Unsupervised learning
This method of learning works on the input data and tries to find correlation and patterns and relationships between the input data and the data that is already available with the machine and then suggests the best course of action based on these findings. This type of learning is usually used in customer segmentation, data extraction and analysis etc.
3. Reinforcement learning
This is a refined version of supervised learning. In this case there is no desired output provided to the machine like in the case of supervised learning and its left to the machine to decide what would be an ideal situation based on which the error message is derived. This is very similar to how humans work as each one of us have our own desired outputs for each action and activity which is dependent on our past experiences and our emotions attached with each of them.
Where can we as business professionals use the growing power of ML to improve efficiency of our various tasks and assignments.
Because of the machine learning capability to analyze and provide data at a much faster pace it enables faster understanding and decision making particularly in situations where the faster we are able to understand live data and take corrective measures the better would be the results.
2. Reduction of Waste
When we didn’t have detailed and structured data the usual methods that were used was to spread the marketing programmes across all likely target groups. This usually leads to a lot of wastage as the materials or programmes need to be send on a very wide base without having any clear idea of their effectiveness. With ML technologies such programmes can be very focused sending this information to the most relevant end customers leading to reduction in waste.
3. Predictive Analytics
Predictive analytics is why we are fed with friend recommendations in various social media platforms after you speak to someone on a call. Or when you are bombarded with emails after you have enquired about a product or searched a product online etc. So, the primary output of any predictive analysis is the targeted variable. The target variable is the feature of a dataset that you want to understand more clearly. It is the variable that the user would want to predict using the rest of the dataset.
4.Structured Content and Material
One of the primary outputs of this part of ML is sentiment variable like the target variable in the earlier case. For example, ML can use the sentiment variable quickly to understand the feelings that a particular content whether written or in video is likely to affect different types of audiences They can also suggest what type of content would maximize results with a particular audience. While this can also be predicted by humans based on their experiences ML provides scale of this activity and this can help an audience without the said experience.
5. Cost Efficiency
Like the many examples that I quoted above the scale and speed of with which ML is able to do all these tasks ensures that the efficiency of scale comes in automatically. It also enables easier customization over a wider range very quickly and hence becomes very much cost efficient.
Machine learning is transforming our daily lives in ways we could only imagine a few years ago. From personalized recommendations on streaming services and e-commerce sites to sophisticated virtual assistants that streamline our tasks, the integration of machine learning in our routine activities is undeniable. It enhances healthcare with predictive diagnostics, optimizes public transport systems, and even aids in climate change research through data analysis.
As these technologies continue to advance, their influence will only grow. The future holds the promise of smarter cities, more intuitive personal devices, and groundbreaking advancements in various fields driven by machine learning. Embracing this technology will be crucial for navigating the complexities of tomorrow, ensuring that we harness its potential for innovation, efficiency, and a better quality of life.
To continue knowing more about how to maximize our effectiveness using technologies like Machine Learning do subscribe to my LinkedIn page, Rejo’s Business Bytes, or my website, rejofrancis.com