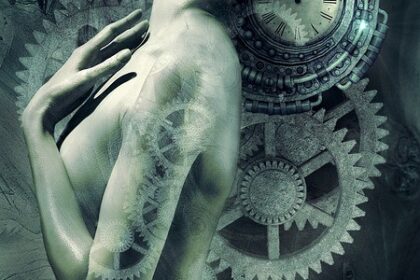
Was a great fan of the Star Trek series when I was a kid and was so fascinated with all the high tech stuff in the show …like data coming up as required right in front of you…you being transported to some distant place…having those small earphones on your ears through which you talked to people back on the mother ship …and the way doctors treated you with implants, portable scans and what not../
That fascination has magnified with the advent of Artificial Intelligence (AI) today.
Today AI already influences us in the form of virtual assistants that are there in most high end smart phones like the Bixby in the Galaxy S8 series or the Siri Assistant in I phones .These virtual assistants are also solving one of the biggest bottlenecks in development of AI, communicating with Machines and software using human languages and symbols. The next step for Artificial intelligence experts is trying to perfect the science of making machines talk back in the same human language at the same pace as we humans do.
Today’s flurry of AI advances wouldn’t have been possible without the confluence of three factors that combined to create the right equation for AI growth: the rise of big data combined with the emergence of powerful graphics processing units (GPUs) for complex computations and the re-emergence of a decades-old AI computation model—deep learning.
It was in 1997 that IBM’s Deep Blue brought real artificial intelligence into the public eye when it bested Chess GM Garry Kasparov in 2 matches.
By 2016, AI had beaten the masters in virtually every game you can think of
From mastering games to contemplating the meaning of life,AI has made rapid stridesin recent years.
The first major challenge that has constantly plagued both AI and the world of computing in general is natural language use.
As the use of chatbots in customer service and other business applications become more prevalent, the ability to recognize the little nuances of human speech is becoming increasingly important. Google has been leading the march towards AI that is indistinguishable from a human being in conversation. Couple of years ago their chatbot was able to have a meaningful debate over the meaning of life. In a few years the keyboard may obsolete as more and more of our devices gain the ability to simply ask us what we’re trying to do.
The next big problem facing AI is the ability to recognize human emotion. A chatbot that can recognize when a person is getting frustrated and adjust its tactics accordingly will go a long way towards improving customer relations.
While the business potential that lies in big data analytics is enormous, the challenge of properly analyzing that data and drawing relevant conclusions is just as big. As AI and machine learning techniques develop, it will completely revolutionize the way we interact with big data. It is creation of this huge data with the development in mobile connectivity and smart phone usage on the go that is speeding up the progress of AI.
One of the methods being adopted by AI researchers is the creation of artificial neural networks. This brings us to different ways in which learning of the neural networks can be optimised.
One method is to live the experiences and replay them in order to guide actions (a process that occurs in a seahorse-shaped brain region called the hippocampus). Similarly, using stored “memories” from its experiences, and feeding these back into its decision-making process during gameplay.
But human brains don’t remember all experiences the same way. They’re biased to remember more emotionally charged events, which are likely to be more important. Future versions of AI should incorporate this kind of biased memory,
Humans know that if you put an object on the table, it’s likely to stay on the table unless the table’s tilted. But nobody writes that in a book—it’s something implicit. Systems don’t have this common-sense capability.
For AI to perceive the same it requires deep learning, deep reasoning and symbolic reasoning
The neural networks of deep learning models require exposure to huge amounts of data to learn a task. Training a neural network to recognize an object, for example, could require feeding it as many as 15 million images. Acquiring relevant datasets of this size can be costly and time-consuming, which slows the pace of training, testing and refining AI systems.
The different types of learning that is being used to superfine- tune AI are as below
· Supervised learning- Where AI learning is actively supervised by humans
· Unsupervised learning- Where help is provided to AI only as and when required so that it makes its own learning without being biased based on human perceptions and experiences
· Reinforcement learning: -As the system takes action in an environment, it’s given rewards if it does good things and penalties if it does bad things. This is much easier to provide in terms of supervision, but it’s still requires supervision,
· Transfer learning: Using a trained model and then, to apply it to a completely new problem, you use just a little bit of training and a little bit of labelled data,
Active learning: The system requests more labeled data only when it needs it. This is a baby step toward unsupervised learning in the sense that the computer is initiating the labeling versus humans just feeding the computer lots and lots of labeled data,”
Just like in nature where Birds flap their wings to fly, but to make humans fly, we had to invent a different type of flying—one that did not occur in nature. And so, similarly, through AI, we’re going to invent many new types of thinking that don’t exist biologically and that are not like human thinking.
Therefore, this intelligence will not replace human thinking, but augment it.
And these will lead to the next evolution where
We will be building computers into our brains by way of “neural lace,” that lays on your brain and bridges it to a computer. It’s the next step beyond even that blending of the digital and physical worlds, as man and machine become one.
So the days where smart phones becomes replaced with humans becoming cyborgs as we saw in Star Trek and Robocop is probably just less than a decade away,